Scala tuple:
Scala tuple is a class and combines a fixed number of items together so that they can be passed around as a whole.
Note:
Example:
Little bag or container you can use to hold things and pass them around.
1)Creating tuples:
Tuples can be created in two ways:
Ex:
To get to know the tuple class:
ii)Creating a tuple with ->:
We can also create tuple with ->, mostly useful at Map collection.
2)Accessing tuple elements:
Accessing tuples in different ways:
We can access tuple elements using an underscore syntax. The first element is accessed with _1, the second element with _2, and so on.
Ex:
ii)Use variable names to access tuple elements:
When referring to a Scala tuple we can also assign names to the elements in the tuple.
Let's we try to do this when returning miscellaneous elements from a method.
Create method, that returns tuple:
Create variables to hold tuple elements:
We can ignore the elements by using an underscore placeholder for the elements you want to ignore.
iii)Iterating over a Scala tuple
As mentioned, a tuple is not a collection; it doesn't descend from any of the collection traits or classes. However, we can treat it a little bit like a collection by using its productIterator method.
iv)The tuple toString method:
The tuple toString method gives you a good representation of a tuple.
Scala tuple is a class and combines a fixed number of items together so that they can be passed around as a whole.
Note:
- Unlike an array or list, a tuple can hold objects with different types but they are also immutable.
- A tuple isn't actually a collection; it's a series of classes named Tuple2, Tuple3, etc., through Tuple22.
Example:
Little bag or container you can use to hold things and pass them around.
1)Creating tuples:
Tuples can be created in two ways:
- Using the enclosing elements in parentheses.
- Creating a tuple with ->
Ex:
val tupleex = (1,"Mano")
To get to know the tuple class:
tupleex.getClass()
ii)Creating a tuple with ->:
We can also create tuple with ->, mostly useful at Map collection.
2)Accessing tuple elements:
Accessing tuples in different ways:
- Using underscore with position
- Use variable names to access tuple elements
- Iterating over a Scala tuple
- The tuple toString method
We can access tuple elements using an underscore syntax. The first element is accessed with _1, the second element with _2, and so on.
Ex:
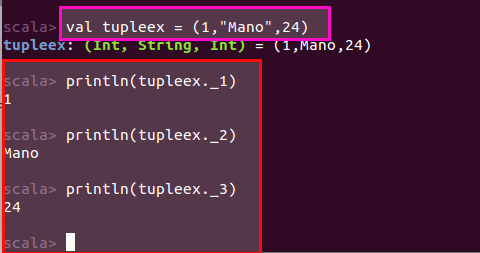
ii)Use variable names to access tuple elements:
When referring to a Scala tuple we can also assign names to the elements in the tuple.
Let's we try to do this when returning miscellaneous elements from a method.
Create method, that returns tuple:
def tuplemeth = (1,"Mano",24)
Create variables to hold tuple elements:
val(id,name,age) = tuplemeth
We can ignore the elements by using an underscore placeholder for the elements you want to ignore.
val(id,name,_) = tuplemeth
iii)Iterating over a Scala tuple
As mentioned, a tuple is not a collection; it doesn't descend from any of the collection traits or classes. However, we can treat it a little bit like a collection by using its productIterator method.
iv)The tuple toString method:
The tuple toString method gives you a good representation of a tuple.